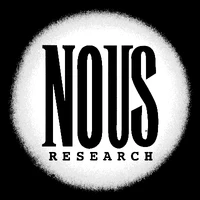
NousResearch/Hermes-2-Pro-Mistral-7B-GGUF
Updated
โข
9.04k
โข
225
Are there any examples or notebooks showing how to use AWQ in LORA fine-tuning a LLM? Or just use AWQ model from huggingface directly? I'm asking as neither the docs nor the Release notes explain anything.
use_dora=True
to your LoraConfig
. Find out more about this method here: https://arxiv.org/abs/2402.09353