yup https://x.com/ylecun/status/1861178764996079752. Would be cool to have a "verified" badge at some point
Clem π€ PRO
clem
AI & ML interests
multi-modal, time-series, biology and chemistry
Recent Activity
liked
a dataset
about 13 hours ago
ylecun/mnist
reacted
to
cfahlgren1's
post
with π
1 day ago
The https://huggingface.co/deepseek-ai/DeepSeek-V3 is very good! I have been playing with it and found it is really good at one-shotting a pretty good landing page.
You can play with it here: https://deepseek-artifacts.vercel.app
All the responses get saved in the https://huggingface.co/datasets/cfahlgren1/react-code-instructions dataset. Hopefully we can build one of the biggest, highest quality frontend datasets on the hub πͺ
Organizations
clem's activity
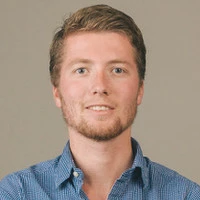
replied to
their
post
about 13 hours ago
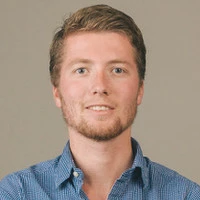
reacted to
cfahlgren1's
post with π
1 day ago
Post
3077
The
deepseek-ai/DeepSeek-V3 is very good! I have been playing with it and found it is really good at one-shotting a pretty good landing page.
You can play with it here: https://deepseek-artifacts.vercel.app
All the responses get saved in the cfahlgren1/react-code-instructions dataset. Hopefully we can build one of the biggest, highest quality frontend datasets on the hub πͺ
You can play with it here: https://deepseek-artifacts.vercel.app
All the responses get saved in the cfahlgren1/react-code-instructions dataset. Hopefully we can build one of the biggest, highest quality frontend datasets on the hub πͺ
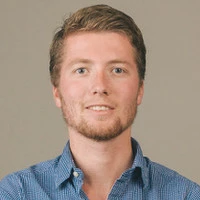
reacted to
csabakecskemeti's
post with πππ€―
1 day ago
Post
2050
The
deepseek-ai/DeepSeek-V3-Base
model has featured today on CNBC tech news. The whale made a splash by using FP8 and shrink the cost of training significantly!
https://youtu.be/NJljq429cGk?si=kgk-ogPTMfJKsaA2
model has featured today on CNBC tech news. The whale made a splash by using FP8 and shrink the cost of training significantly!
https://youtu.be/NJljq429cGk?si=kgk-ogPTMfJKsaA2
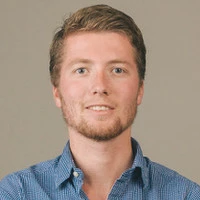
reacted to
sequelbox's
post with ππ
1 day ago
Post
2048
Check out the early preview of the upcoming Tachibana-QVQ dataset: code-reasoning and code-instruct data generated with
Qwen/QVQ-72B-Preview
Link here: sequelbox/Tachibana-QVQ-PREVIEW
more to come :)
Link here: sequelbox/Tachibana-QVQ-PREVIEW
more to come :)
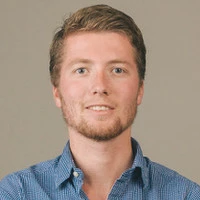
reacted to
merve's
post with β€οΈππ₯
1 day ago
Post
3894
supercharge your LLM apps with smolagents π₯
however cool your LLM is, without being agentic it can only go so far
enter smolagents: a new agent library by Hugging Face to make the LLM write code, do analysis and automate boring stuff!
Here's our blog for you to get started https://huggingface.co/blog/smolagents
however cool your LLM is, without being agentic it can only go so far
enter smolagents: a new agent library by Hugging Face to make the LLM write code, do analysis and automate boring stuff!
Here's our blog for you to get started https://huggingface.co/blog/smolagents
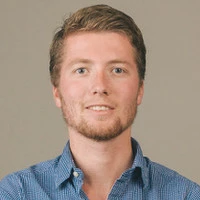
reacted to
tomaarsen's
post with ππ₯β€οΈ
1 day ago
Post
2395
That didn't take long! Nomic AI has finetuned the new ModernBERT-base encoder model into a strong embedding model for search, classification, clustering and more!
Details:
π€ Based on ModernBERT-base with 149M parameters.
π Outperforms both nomic-embed-text-v1 and nomic-embed-text-v1.5 on MTEB!
ποΈ Immediate FA2 and unpacking support for super efficient inference.
πͺ Trained with Matryoshka support, i.e. 2 valid output dimensionalities: 768 and 256.
β‘οΈ Maximum sequence length of 8192 tokens!
2οΈβ£ Trained in 2 stages: unsupervised contrastive data -> high quality labeled datasets.
β Integrated in Sentence Transformers, Transformers, LangChain, LlamaIndex, Haystack, etc.
ποΈ Apache 2.0 licensed: fully commercially permissible
Try it out here: nomic-ai/modernbert-embed-base
Very nice work by Zach Nussbaum and colleagues at Nomic AI.
Details:
π€ Based on ModernBERT-base with 149M parameters.
π Outperforms both nomic-embed-text-v1 and nomic-embed-text-v1.5 on MTEB!
ποΈ Immediate FA2 and unpacking support for super efficient inference.
πͺ Trained with Matryoshka support, i.e. 2 valid output dimensionalities: 768 and 256.
β‘οΈ Maximum sequence length of 8192 tokens!
2οΈβ£ Trained in 2 stages: unsupervised contrastive data -> high quality labeled datasets.
β Integrated in Sentence Transformers, Transformers, LangChain, LlamaIndex, Haystack, etc.
ποΈ Apache 2.0 licensed: fully commercially permissible
Try it out here: nomic-ai/modernbert-embed-base
Very nice work by Zach Nussbaum and colleagues at Nomic AI.
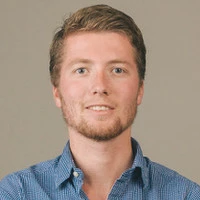
reacted to
singhsidhukuldeep's
post with β€οΈπ€―
1 day ago
Post
1539
Excited to share insights from Walmart's groundbreaking semantic search system that revolutionizes e-commerce product discovery!
The team at Walmart Global Technology(the team that I am a part of π¬) has developed a hybrid retrieval system that combines traditional inverted index search with neural embedding-based search to tackle the challenging problem of tail queries in e-commerce.
Key Technical Highlights:
β’ The system uses a two-tower BERT architecture where one tower processes queries and another processes product information, generating dense vector representations for semantic matching.
β’ Product information is enriched by combining titles with key attributes like category, brand, color, and gender using special prefix tokens to help the model distinguish different attribute types.
β’ The neural model leverages DistilBERT with 6 layers and projects the 768-dimensional embeddings down to 256 dimensions using a linear layer, achieving optimal performance while reducing storage and computation costs.
β’ To improve model training, they implemented innovative negative sampling techniques combining product category matching and token overlap filtering to identify challenging negative examples.
Production Implementation Details:
β’ The system uses a managed ANN (Approximate Nearest Neighbor) service to enable fast retrieval, achieving 99% recall@20 with just 13ms latency.
β’ Query embeddings are cached with preset TTL (Time-To-Live) to reduce latency and costs in production.
β’ The model is exported to ONNX format and served in Java, with custom optimizations like fixed input shapes and GPU acceleration using NVIDIA T4 processors.
Results:
The system showed significant improvements in both offline metrics and live experiments, with:
- +2.84% improvement in NDCG@10 for human evaluation
- +0.54% lift in Add-to-Cart rates in live A/B testing
This is a fantastic example of how modern NLP techniques can be successfully deployed at scale to solve real-world e-
The team at Walmart Global Technology(the team that I am a part of π¬) has developed a hybrid retrieval system that combines traditional inverted index search with neural embedding-based search to tackle the challenging problem of tail queries in e-commerce.
Key Technical Highlights:
β’ The system uses a two-tower BERT architecture where one tower processes queries and another processes product information, generating dense vector representations for semantic matching.
β’ Product information is enriched by combining titles with key attributes like category, brand, color, and gender using special prefix tokens to help the model distinguish different attribute types.
β’ The neural model leverages DistilBERT with 6 layers and projects the 768-dimensional embeddings down to 256 dimensions using a linear layer, achieving optimal performance while reducing storage and computation costs.
β’ To improve model training, they implemented innovative negative sampling techniques combining product category matching and token overlap filtering to identify challenging negative examples.
Production Implementation Details:
β’ The system uses a managed ANN (Approximate Nearest Neighbor) service to enable fast retrieval, achieving 99% recall@20 with just 13ms latency.
β’ Query embeddings are cached with preset TTL (Time-To-Live) to reduce latency and costs in production.
β’ The model is exported to ONNX format and served in Java, with custom optimizations like fixed input shapes and GPU acceleration using NVIDIA T4 processors.
Results:
The system showed significant improvements in both offline metrics and live experiments, with:
- +2.84% improvement in NDCG@10 for human evaluation
- +0.54% lift in Add-to-Cart rates in live A/B testing
This is a fantastic example of how modern NLP techniques can be successfully deployed at scale to solve real-world e-
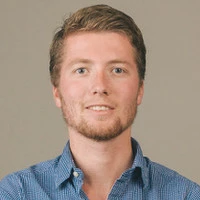
reacted to
DamarJati's
post with πββ€οΈ
1 day ago
Post
2033
Happy New Year 2025 π€
For the Huggingface community.
For the Huggingface community.
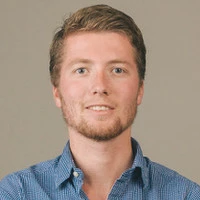
reacted to
prithivMLmods's
post with β€οΈπ₯
1 day ago
Post
3014
Triangulum Catalogued π₯π«
π―Triangulum is a collection of pretrained and instruction-tuned generative models, designed for multilingual applications. These models are trained using synthetic datasets based on long chains of thought, enabling them to perform complex reasoning tasks effectively.
+ Triangulum-10B : prithivMLmods/Triangulum-10B
+ Quants : prithivMLmods/Triangulum-10B-GGUF
+ Triangulum-5B : prithivMLmods/Triangulum-5B
+ Quants : prithivMLmods/Triangulum-5B-GGUF
+ Triangulum-1B : prithivMLmods/Triangulum-1B
+ Quants : prithivMLmods/Triangulum-1B-GGUF
π―Triangulum is a collection of pretrained and instruction-tuned generative models, designed for multilingual applications. These models are trained using synthetic datasets based on long chains of thought, enabling them to perform complex reasoning tasks effectively.
+ Triangulum-10B : prithivMLmods/Triangulum-10B
+ Quants : prithivMLmods/Triangulum-10B-GGUF
+ Triangulum-5B : prithivMLmods/Triangulum-5B
+ Quants : prithivMLmods/Triangulum-5B-GGUF
+ Triangulum-1B : prithivMLmods/Triangulum-1B
+ Quants : prithivMLmods/Triangulum-1B-GGUF