Kuldeep Singh Sidhu
singhsidhukuldeep
AI & ML interests
😃 TOP 3 on HuggingFace for posts 🤗 Seeking contributors for a completely open-source 🚀 Data Science platform! singhsidhukuldeep.github.io
Recent Activity
posted
an
update
about 10 hours ago
Groundbreaking Research Alert: Rethinking RAG with Cache-Augmented Generation (CAG)
Researchers from National Chengchi University and Academia Sinica have introduced a paradigm-shifting approach that challenges the conventional wisdom of Retrieval-Augmented Generation (RAG).
Instead of the traditional retrieve-then-generate pipeline, their innovative Cache-Augmented Generation (CAG) framework preloads documents and precomputes key-value caches, eliminating the need for real-time retrieval during inference.
Technical Deep Dive:
- CAG preloads external knowledge and precomputes KV caches, storing them for future use
- The system processes documents only once, regardless of subsequent query volume
- During inference, it loads the precomputed cache alongside user queries, enabling rapid response generation
- The cache reset mechanism allows efficient handling of multiple inference sessions through strategic token truncation
Performance Highlights:
- Achieved superior BERTScore metrics compared to both sparse and dense retrieval RAG systems
- Demonstrated up to 40x faster generation times compared to traditional approaches
- Particularly effective with both SQuAD and HotPotQA datasets, showing robust performance across different knowledge tasks
Why This Matters:
The approach significantly reduces system complexity, eliminates retrieval latency, and mitigates common RAG pipeline errors. As LLMs continue evolving with expanded context windows, this methodology becomes increasingly relevant for knowledge-intensive applications.
posted
an
update
4 days ago
Excited to share insights from Walmart's groundbreaking semantic search system that revolutionizes e-commerce product discovery!
The team at Walmart Global Technology(the team that I am a part of 😬) has developed a hybrid retrieval system that combines traditional inverted index search with neural embedding-based search to tackle the challenging problem of tail queries in e-commerce.
Key Technical Highlights:
• The system uses a two-tower BERT architecture where one tower processes queries and another processes product information, generating dense vector representations for semantic matching.
• Product information is enriched by combining titles with key attributes like category, brand, color, and gender using special prefix tokens to help the model distinguish different attribute types.
• The neural model leverages DistilBERT with 6 layers and projects the 768-dimensional embeddings down to 256 dimensions using a linear layer, achieving optimal performance while reducing storage and computation costs.
• To improve model training, they implemented innovative negative sampling techniques combining product category matching and token overlap filtering to identify challenging negative examples.
Production Implementation Details:
• The system uses a managed ANN (Approximate Nearest Neighbor) service to enable fast retrieval, achieving 99% recall@20 with just 13ms latency.
• Query embeddings are cached with preset TTL (Time-To-Live) to reduce latency and costs in production.
• The model is exported to ONNX format and served in Java, with custom optimizations like fixed input shapes and GPU acceleration using NVIDIA T4 processors.
Results:
The system showed significant improvements in both offline metrics and live experiments, with:
- +2.84% improvement in NDCG@10 for human evaluation
- +0.54% lift in Add-to-Cart rates in live A/B testing
This is a fantastic example of how modern NLP techniques can be successfully deployed at scale to solve real-world e-
posted
an
update
7 days ago
Groundbreaking Research Alert: Revolutionizing Document Ranking with Long-Context LLMs
Researchers from Renmin University of China and Baidu Inc . have introduced a novel approach to document ranking that challenges conventional sliding window methods. Their work demonstrates how long-context Large Language Models can process up to 100 documents simultaneously, achieving superior performance while reducing API costs by 50%.
Key Technical Innovations:
- Full ranking strategy enables processing all passages in a single inference
- Multi-pass sliding window approach for comprehensive listwise label construction
- Importance-aware learning objective that prioritizes top-ranked passage IDs
- Support for context lengths up to 128k tokens using models like LLaMA 3.1-8B-Instruct
Performance Highlights:
- 2.2 point improvement in NDCG@10 metrics
- 29.3% reduction in latency compared to traditional methods
- Significant API cost savings through elimination of redundant passage processing
Under the hood, the system leverages advanced long-context LLMs to perform global interactions among passages, enabling more nuanced relevance assessment. The architecture incorporates a novel importance-aware loss function that assigns differential weights based on passage ranking positions.
The research team's implementation demonstrated remarkable versatility across multiple datasets, including TREC DL and BEIR benchmarks. Their fine-tuned model, RankMistral, showcases the practical viability of full ranking approaches in production environments.
This advancement marks a significant step forward in information retrieval systems, offering both improved accuracy and computational efficiency. The implications for search engines and content recommendation systems are substantial.
Organizations
singhsidhukuldeep's activity
Update Request
2
#2 opened about 2 months ago
by
singhsidhukuldeep
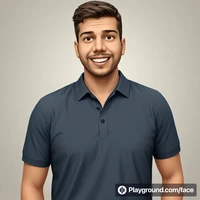
The model can be started using vllm, but no dialogue is possible.
3
#2 opened 5 months ago
by
SongXiaoMao
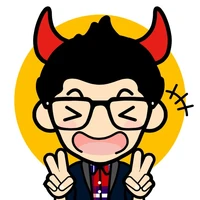
Adding chat_template to tokenizer_config.json file
1
#3 opened 5 months ago
by
singhsidhukuldeep
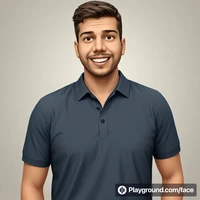
Script request
3
#1 opened 5 months ago
by
singhsidhukuldeep
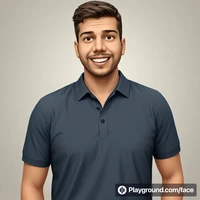
Requesting script
#1 opened 5 months ago
by
singhsidhukuldeep
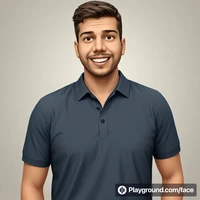